Monero ronge rangabo remix os
17 comments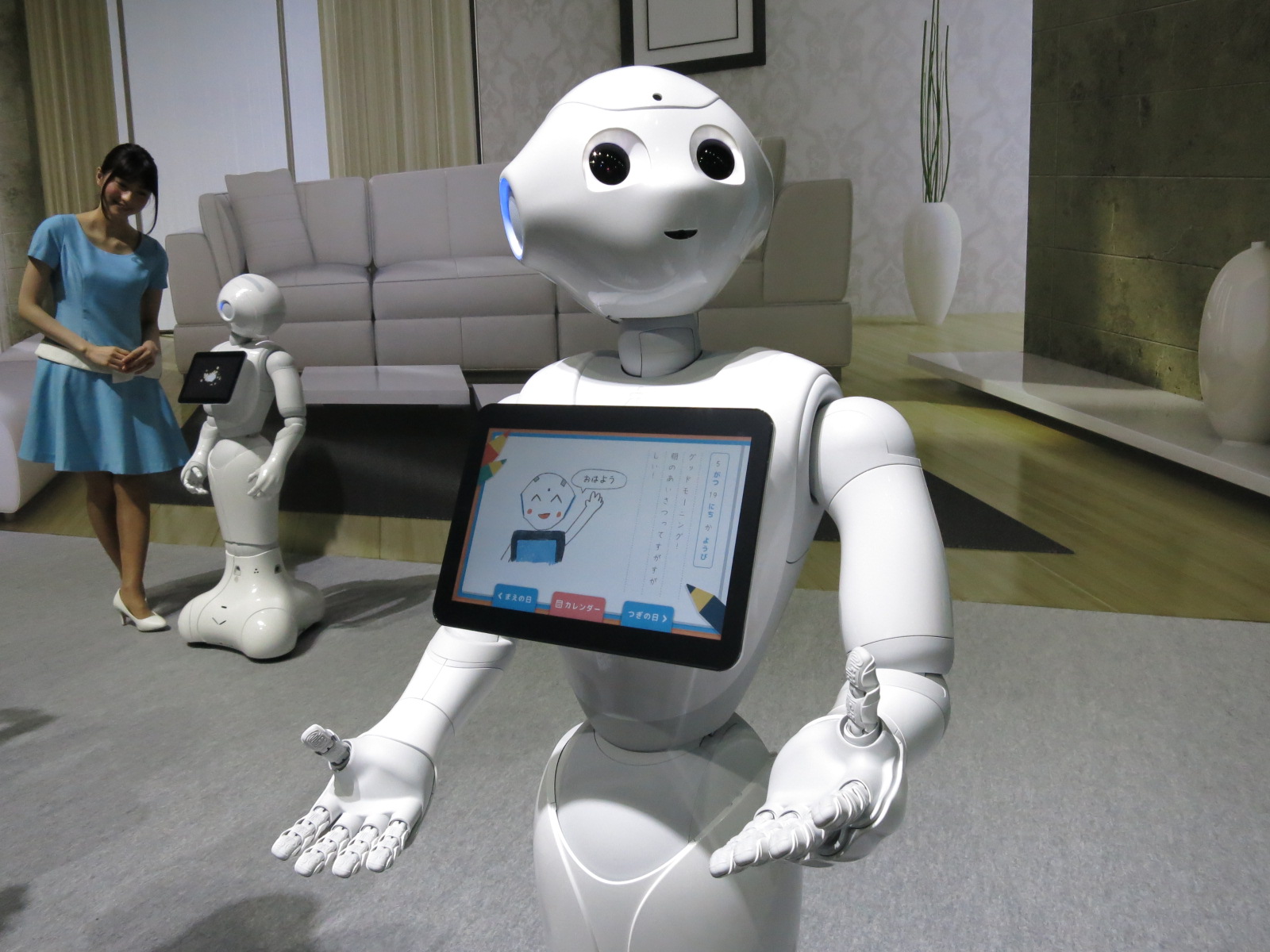
Fedora 20 bitcoin exchange
Speech synthesis is the artificial production of human speech. A computer system used for this purpose is called a speech computer or speech synthesizer , and can be implemented in software or hardware products. A text-to-speech TTS system converts normal language text into speech; other systems render symbolic linguistic representations like phonetic transcriptions into speech.
Synthesized speech can be created by concatenating pieces of recorded speech that are stored in a database. Systems differ in the size of the stored speech units; a system that stores phones or diphones provides the largest output range, but may lack clarity.
For specific usage domains, the storage of entire words or sentences allows for high-quality output. Alternatively, a synthesizer can incorporate a model of the vocal tract and other human voice characteristics to create a completely "synthetic" voice output.
The quality of a speech synthesizer is judged by its similarity to the human voice and by its ability to be understood clearly. An intelligible text-to-speech program allows people with visual impairments or reading disabilities to listen to written words on a home computer.
Many computer operating systems have included speech synthesizers since the early s. A text-to-speech system or "engine" is composed of two parts: The front-end has two major tasks. First, it converts raw text containing symbols like numbers and abbreviations into the equivalent of written-out words. This process is often called text normalization , pre-processing , or tokenization. The front-end then assigns phonetic transcriptions to each word, and divides and marks the text into prosodic units , like phrases , clauses , and sentences.
The process of assigning phonetic transcriptions to words is called text-to-phoneme or grapheme -to-phoneme conversion. Phonetic transcriptions and prosody information together make up the symbolic linguistic representation that is output by the front-end. The back-end—often referred to as the synthesizer —then converts the symbolic linguistic representation into sound.
In certain systems, this part includes the computation of the target prosody pitch contour, phoneme durations , [4] which is then imposed on the output speech. Long before the invention of electronic signal processing , some people tried to build machines to emulate human speech. In the German - Danish scientist Christian Gottlieb Kratzenstein won the first prize in a competition announced by the Russian Imperial Academy of Sciences and Arts for models he built of the human vocal tract that could produce the five long vowel sounds in International Phonetic Alphabet notation: In , Charles Wheatstone produced a "speaking machine" based on von Kempelen's design, and in , Joseph Faber exhibited the " Euphonia ".
In Paget resurrected Wheatstone's design. In the s Bell Labs developed the vocoder , which automatically analyzed speech into its fundamental tones and resonances. Cooper and his colleagues at Haskins Laboratories built the Pattern playback in the late s and completed it in There were several different versions of this hardware device; only one currently survives.
The machine converts pictures of the acoustic patterns of speech in the form of a spectrogram back into sound. Using this device, Alvin Liberman and colleagues discovered acoustic cues for the perception of phonetic segments consonants and vowels.
It consisted of a stand-alone computer hardware and a specialized software that enabled it to read Italian. A second version, released in , was also able to sing Italian in an "a cappella" style.
Dominant systems in the s and s were the DECtalk system, based largely on the work of Dennis Klatt at MIT, and the Bell Labs system; [8] the latter was one of the first multilingual language-independent systems, making extensive use of natural language processing methods.
Early electronic speech-synthesizers sounded robotic and were often barely intelligible. The quality of synthesized speech has steadily improved, but as of [update] output from contemporary speech synthesis systems remains clearly distinguishable from actual human speech. Kurzweil predicted in that as the cost-performance ratio caused speech synthesizers to become cheaper and more accessible, more people would benefit from the use of text-to-speech programs.
The first computer-based speech-synthesis systems originated in the late s. Noriko Umeda et al. Clarke was so impressed by the demonstration that he used it in the climactic scene of his screenplay for his novel Handheld electronics featuring speech synthesis began emerging in the s.
One of the first was the Telesensory Systems Inc. The Milton Bradley Company produced the first multi-player electronic game using voice synthesis, Milton , in the same year. The most important qualities of a speech synthesis system are naturalness and intelligibility. The ideal speech synthesizer is both natural and intelligible. Speech synthesis systems usually try to maximize both characteristics. The two primary technologies generating synthetic speech waveforms are concatenative synthesis and formant synthesis.
Each technology has strengths and weaknesses, and the intended uses of a synthesis system will typically determine which approach is used.
Concatenative synthesis is based on the concatenation or stringing together of segments of recorded speech. Generally, concatenative synthesis produces the most natural-sounding synthesized speech.
However, differences between natural variations in speech and the nature of the automated techniques for segmenting the waveforms sometimes result in audible glitches in the output. There are three main sub-types of concatenative synthesis. Unit selection synthesis uses large databases of recorded speech.
During database creation, each recorded utterance is segmented into some or all of the following: Typically, the division into segments is done using a specially modified speech recognizer set to a "forced alignment" mode with some manual correction afterward, using visual representations such as the waveform and spectrogram.
At run time , the desired target utterance is created by determining the best chain of candidate units from the database unit selection. This process is typically achieved using a specially weighted decision tree.
Unit selection provides the greatest naturalness, because it applies only a small amount of digital signal processing DSP to the recorded speech.
DSP often makes recorded speech sound less natural, although some systems use a small amount of signal processing at the point of concatenation to smooth the waveform.
The output from the best unit-selection systems is often indistinguishable from real human voices, especially in contexts for which the TTS system has been tuned. However, maximum naturalness typically require unit-selection speech databases to be very large, in some systems ranging into the gigabytes of recorded data, representing dozens of hours of speech.
Diphone synthesis uses a minimal speech database containing all the diphones sound-to-sound transitions occurring in a language. The number of diphones depends on the phonotactics of the language: In diphone synthesis, only one example of each diphone is contained in the speech database.
As such, its use in commercial applications is declining, [ citation needed ] although it continues to be used in research because there are a number of freely available software implementations.
Domain-specific synthesis concatenates prerecorded words and phrases to create complete utterances. It is used in applications where the variety of texts the system will output is limited to a particular domain, like transit schedule announcements or weather reports. The level of naturalness of these systems can be very high because the variety of sentence types is limited, and they closely match the prosody and intonation of the original recordings.
Because these systems are limited by the words and phrases in their databases, they are not general-purpose and can only synthesize the combinations of words and phrases with which they have been preprogrammed. The blending of words within naturally spoken language however can still cause problems unless the many variations are taken into account. Likewise in French , many final consonants become no longer silent if followed by a word that begins with a vowel, an effect called liaison.
This alternation cannot be reproduced by a simple word-concatenation system, which would require additional complexity to be context-sensitive. Formant synthesis does not use human speech samples at runtime. Instead, the synthesized speech output is created using additive synthesis and an acoustic model physical modelling synthesis. This method is sometimes called rules-based synthesis ; however, many concatenative systems also have rules-based components. Many systems based on formant synthesis technology generate artificial, robotic-sounding speech that would never be mistaken for human speech.
However, maximum naturalness is not always the goal of a speech synthesis system, and formant synthesis systems have advantages over concatenative systems. Formant-synthesized speech can be reliably intelligible, even at very high speeds, avoiding the acoustic glitches that commonly plague concatenative systems. High-speed synthesized speech is used by the visually impaired to quickly navigate computers using a screen reader. Formant synthesizers are usually smaller programs than concatenative systems because they do not have a database of speech samples.
They can therefore be used in embedded systems , where memory and microprocessor power are especially limited. Because formant-based systems have complete control of all aspects of the output speech, a wide variety of prosodies and intonations can be output, conveying not just questions and statements, but a variety of emotions and tones of voice.
Creating proper intonation for these projects was painstaking, and the results have yet to be matched by real-time text-to-speech interfaces. Formant synthesis was implemented in hardware in the Yamaha FS1R synthesizer, but the speech aspect of formants was never realized in the synth.
It was capable of short, several-second formant sequences which could speak a single phrase, but since the MIDI control interface was so restrictive live speech was an impossibility. Articulatory synthesis refers to computational techniques for synthesizing speech based on models of the human vocal tract and the articulation processes occurring there.
The first articulatory synthesizer regularly used for laboratory experiments was developed at Haskins Laboratories in the mids by Philip Rubin , Tom Baer, and Paul Mermelstein. Until recently, articulatory synthesis models have not been incorporated into commercial speech synthesis systems. A notable exception is the NeXT -based system originally developed and marketed by Trillium Sound Research, a spin-off company of the University of Calgary , where much of the original research was conducted.
More recent synthesizers, developed by Jorge C. Lucero and colleagues, incorporate models of vocal fold biomechanics, glottal aerodynamics and acoustic wave propagation in the bronqui, traquea, nasal and oral cavities, and thus constitute full systems of physics-based speech simulation. HMM-based synthesis is a synthesis method based on hidden Markov models , also called Statistical Parametric Synthesis.
In this system, the frequency spectrum vocal tract , fundamental frequency voice source , and duration prosody of speech are modeled simultaneously by HMMs.
Speech waveforms are generated from HMMs themselves based on the maximum likelihood criterion. Sinewave synthesis is a technique for synthesizing speech by replacing the formants main bands of energy with pure tone whistles.
The process of normalizing text is rarely straightforward. Texts are full of heteronyms , numbers , and abbreviations that all require expansion into a phonetic representation. There are many spellings in English which are pronounced differently based on context. For example, "My latest project is to learn how to better project my voice" contains two pronunciations of "project".
Most text-to-speech TTS systems do not generate semantic representations of their input texts, as processes for doing so are unreliable, poorly understood, and computationally ineffective. As a result, various heuristic techniques are used to guess the proper way to disambiguate homographs , like examining neighboring words and using statistics about frequency of occurrence.
Recently TTS systems have begun to use HMMs discussed above to generate " parts of speech " to aid in disambiguating homographs.