Daniel kraft bitcoin
13 comments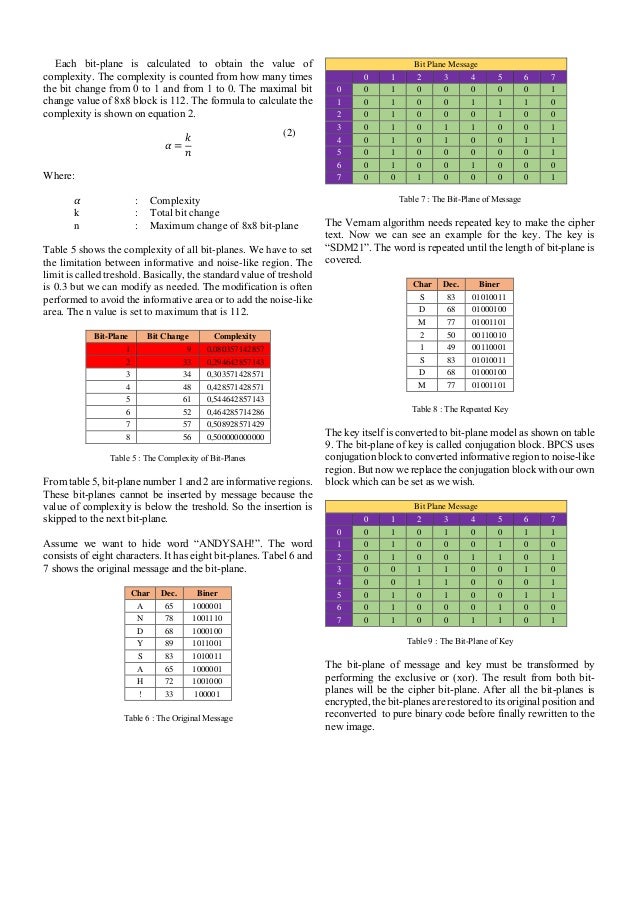
Bitcoin account trading
Steganography is defined as "the art and science of writing hidden messages such that no one apart from the intended recipient knows of the existence of the message" en. To test its viability, I implemented the technique in Python. Bit-Plane Complexity Segmentation, a lossy image-compression technique first proposed at the Kyushu Institute of Technology, lets you embed large amounts of data in images.
But to do so, you need visual cryptography to decompose a message in two shares with a highly random character. Generally, visual cryptography is considered a visual form of secret sharing; see Doug Stinson's article "Visual Cryptography and Threshold Schemes" www. In its simplest form, a 2,2 visual cryptography scheme "splits" the original image into two "shadow images" called "shares. Any share contains uniformly distributed random black-and-white pixels.
By analyzing only a single share, you can't obtain information about the original image, no matter how much computing power or analysis method is used. The whole point of visual cryptography is that in the decryption process, the original image has to be visually reconstructed. Each share is printed on a separate transparency and passed to a participant at the scheme. When the two participants come together, the secret can simply and theoretically instantaneously be reconstructed by stacking the two transparencies.
Figure 1 is an example of the visual cryptography scheme implemented by the application. Bit-Plane Complexity Segmentation BPCS relies on the fact that the human visual system is sensitive to patterns, but unable to identify random white noise.
Therefore, if you want to implement a BCPS algorithm, you divide the image into regions and calculate the complexity of these regions. Any region with complexity above a certain threshold can be replaced with embedded data. This technique works on bit true-color or 8-bit grayscale images. It doesn't work on paletted images; small changes in a pixel value might have drastic effects on the color of the pixel in the paletted image. Kawaguchy and Eason citeseer. Most of the LSBs just look like random noise Figure 2.
Following the separation of the image in bit-planes, every bit-plane is decomposed in square regions and the complexity of the regions is calculated. There is no general definition for the complexity of a binary image. To define a coherent scale of complexities, you normalize this figure such that one plain color has a complexity of 0 and the checkerboard pattern the most complex possible region has a complexity of 1.
Any region in any bit-plane with a complexity above a chosen threshold is considered random noise and replaced by 8 bytes of data. It is still possible that an embedded block will not have a complexity above the threshold value.
In this case, the conjugate of the block must be taken. The conjugate of a binary image is obtained by XOR ing the image with the checkerboard pattern. Obviously, the original data can be remade by XOR ing the new image with the checkerboard pattern again.
If necessary, the conjugate is calculated,and you need a "flag pixel" to mark the region as "conjugated. In the decryption process as in a puzzle , you have to detect any piece of embedded data and put it back in place. To properly identify every square, I mark each with a sequence number.
The sequence number is an integer number binary representation. Because the proposed embedding scheme is "blind" that is, no prior knowledge of the image or other information is needed to extract the embedded message , the additional information consisting of the conjugate flag and sequence number has to be written back into the image, together with the embedding data.
To win space for the supplementary information that mustaccompany every square, you can border any square with a row and a column; the information could be organized as in Figure 3. The algorithm doesn't work if you embed more squares than can be marked with a sequence number. From this perspective, the maximum amount of information that can be embedded is , bytes, or KB of data. This is because the lossy compression algorithms are likely to discard the LSB and the most important details are usually in the middle of the image.
A bit true-color image consists of red, green, and blue RGB. The human visual system seems sensitive to green variations and less sensitive to blue ones. Therefore, I adopt the following order in embedding data: I start with the MSB of every constituent color, then move to the next plane until all the embedding information is written.
The order of the color components is blue, red, green. Though the embedding scheme is known for all images, it does not have to be communicated to the receiving party. The receiver only has to divide the image into color components, then bit-planes and regions. Afterwards, all regions having a complexity above the predefined threshold are checked for data. If a region has errors and is not identified by its complexity or if the sequence number was altered , the receiver misses the block.
But it is possible to guess which region should contain data see Listing Seven, available at http: A possible scenario for the proposed model involves a "dealer" and "participants" to the scheme.
The dealer chooses a secret message represented as a binary black-and-white image and applies a 2,2 visual-cryptography scheme on the secret message, obtaining the two corresponding shares. Every share is individually embedded into an 8-bit grayscale image traditionally called a "vessel" using the modified BPCS scheme.
Finally, the dealer electronically sends the images with embedded data to the participants. Participants process the received image also based on the modified BPCS scheme , obtain the embedded share as a binary image, and print the binary image on a transparency.
As soon as the participants come together, they can visually reconstruct the secret message by carefully superimposing the two transparencies. This model follows the block diagram in Figure 4. Figure 5 presents a general view considering the modality the data is embedded into the vessels. PIL adds image-processing capabilities to Python and processes a range of image formats. There is a common convention in processing binary black-and-white images: If any black pixel in the image is denoted by 1 and any white pixel is 0, you can "transform" any binary black-and-white image into a binary matrix.
This is the approach used in my application. In the embedding process, you select squares from a larger matrix a share in the model and write back squares into some other matrix normally a bit-plane ; see Listings One and Two, respectively. The algorithm to calculate the complexity of a region in a bit-plane can be implemented as in Listing Three.
To ensure the "blind" character of the embedding scheme, you have to provide additional information like sequence number and the flag for the conjugated areas to any square read from a share.
The additional information is written back into the vessel, together with the square of embedding data. The required space is obtained by bordering the square with one row and one column. Though generally defined, the functions in Listings Four a and b can perform the bordering as well. The program also provides other tools for tasks such as generating a random binary matrix or checkerboard pattern, initializing log files, defining some standard directories, and the like.
All these are implemented in a utils package. The application has compression, embedding, and extraction modules. All components work on 8-bit gray-level or bit true-color images. I focus on the 8-bit images because the processing of the true-color images only involves this extension: PIL lets you switch between different pixel representations of an image using the convert function.
In terms of converted images, there are only two accepted formats: This lets you convert input images in RGB format like this:. After a successful conversion, the image object is in RGB format. For such objects, PIL offers a method that returns a tuple containing the individual image components "bands" in the PIL documentation. This means you can say:. The red , green , blue objects this time are 8-bit grayscale images.
All three modules of the application are implemented in a separate class called image. Compression, which is based on BPCS, only determines image regions with a complexity above a determined threshold. These regions are overwritten with white color. The embedding module uses the modified BPCS scheme.
The module performs the bordering of the initial square, correspondingly inserting the sequence numbers and the "conjugated flag" if needed see Listing Six, available at www.
The function starts with a given sequence number and returns the last sequence number it used. This is because of the embedding scenario itself: During the process, you have to move from one bit-plane to another, and, in the case of true-color images, from one color component to another.
So the function has to "know" what the current sequence number is and return the sequence number it finished with for the next iteration. The extraction module checks the complexity of any region in the image and searches for embedded data. The application also tries to determine if the complete chain of sequence numbers was extracted; if not, it reports the missing squares.
As in Table 1, the only matrices that should exist in a share are the diagonal ones. As the embedding function, the extraction function starts with an initial sequence number and returns the next sequence number when finished see Listing Seven, available at www.